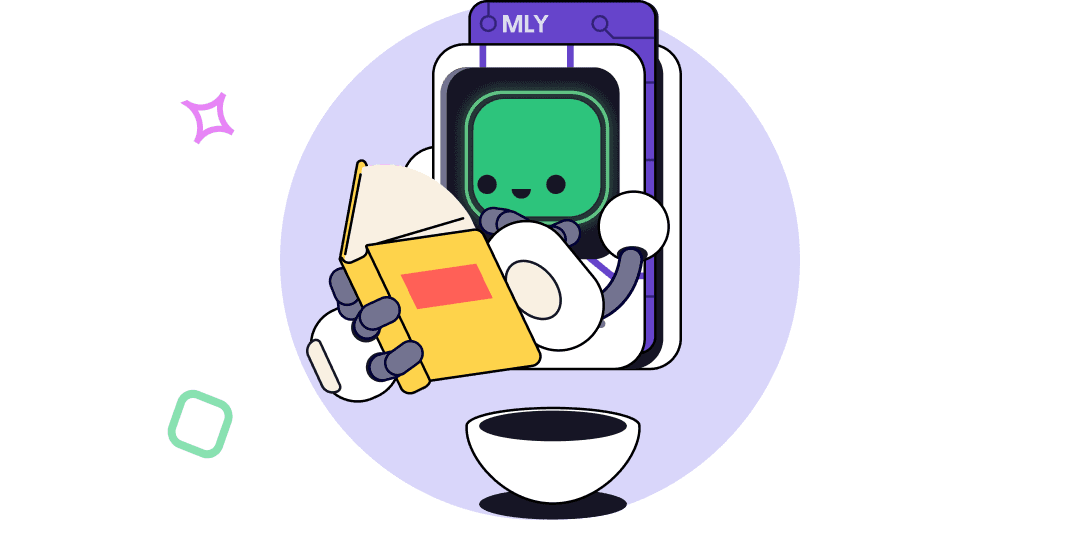
Qualitative Analysis Can Be Difficult, Costly, and Time-Consuming
Whether it's manual human-read analysis or thematic coding with text analytics, qualitative analysis of student comments is often disregarded due to its resource-intensive nature. If that happens, you may be ignoring crucial insights about the student experience.